Publication
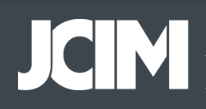
D. Warszycki
,
Ł. Struski
,
M. Śmieja
,
R. Kafel
,
and
R. Kurczab
Pharmacoprint: A Combination of a Pharmacophore Fingerprint and Artificial Intelligence as a Tool for Computer-Aided Drug Design
Journal of Chemical Information and Modeling, 61, 5054-5065, 2021
URL, DOI , RIS, BibTex
Pharmacoprint: A Combination of a Pharmacophore Fingerprint and Artificial Intelligence as a Tool for Computer-Aided Drug Design
Journal of Chemical Information and Modeling, 61, 5054-5065, 2021
URL, DOI , RIS, BibTex
Abstract
Structural fingerprints and pharmacophore modeling are methodologies that have been used for at least 2 decades in various fields of cheminformatics, from similarity searching to machine learning (ML). Advances in in silico techniques consequently led to combining both these methodologies into a new approach known as the pharmacophore fingerprint. Herein, we propose a high-resolution, pharmacophore fingerprint called Pharmacoprint that encodes the presence, types, and relationships between pharmacophore features of a molecule. Pharmacoprint was evaluated in classification experiments by using ML algorithms (logistic regression, support vector machines, linear support vector machines, and neural networks) and outperformed other popular molecular fingerprints (i.e., ECFP4, Estate, MACCS, PubChem, Substructure, Klekota-Roth, CDK, Extended, and GraphOnly) and the ChemAxon pharmacophoric features fingerprint. Pharmacoprint consisted of 39 973 bits; several methods were applied for dimensionality reduction, and the best algorithm not only reduced the length of the bit string but also improved the efficiency of the ML tests. Further optimization allowed us to define the best parameter settings for using Pharmacoprint in discrimination tests and for maximizing statistical parameters. Finally, Pharmacoprint generated for three-dimensional (3D) structures with defined hydrogens as input data was applied to neural networks with a supervised autoencoder for selecting the most important bits and allowed us to maximize the Matthews correlation coefficient up to 0.962. The results show the potential of Pharmacoprint as a new, perspective tool for computer-aided drug design.
Reference
@article{Warszycki2021,
author = "D. Warszycki and Ł. Struski and M. Śmieja and R. Kafel and R. Kurczab",
title = "Pharmacoprint: A Combination of a Pharmacophore Fingerprint and Artificial Intelligence as a Tool for Computer-Aided Drug Design",
year = 2021,
journal = "Journal of Chemical Information and Modeling",
volume = 61,
pages = "5054-5065",
doi = "10.1021/acs.jcim.1c00589",
url = "https://pubs.acs.org/doi/10.1021/acs.jcim.1c00589"
}